Use of Big Data in ECHO
SmartResilience project is finalized to study vulnerability, smartness and resilience of modern critical infrastructures when exposed to extreme threats which are becoming increasingly smarter.
The project envisages addressing the above challenges in several steps. (#1) By identifying existing indicators suitable for assessing resilience of SCIs (#2) By identifying new smart resilience indicators including those from Big Data (#3) By developing, a new advanced resilience assessment methodology based on smart RIs and the resilience indicators cube, including the resilience matrix (#4) By developing the interactive SCI Dashboard tool (#5) By applying the methodology/tools in 8 case studies, integrated under one virtual, smart-city-like, European case study. The SCIs considered (in 8 European countries!) deal with energy, transportation, health, and water. (http://smartresilience.eu-vri.eu/sites/default/files/publications/SmartResD5.1.pdf)
In the SmartResilience project, we use two different approaches for obtaining indicators. The first is the use of conventional indicators in a top-down manner, and the second is bottom-up use of indicators from big data or open data sources. In the top-down approach, we will make sure that most/all relevant issues are covered with relevant conventional indicators. This means that indicators from big data or open data sources will be additional and especially useful for capturing smart technology issues that supplements the conventional indicators. In this way, we are not dependent on the big data or open data sources for every dimension and every issue. Moreover, some cities or critical infrastructures may not be especially advanced with respect to use of smart technologies, but still need to be assigned a resilience level.
Analysis of big data helps in deriving indicators through network or complex systems approaches. What makes the network approach attractive is that it provides a formal and quantitative language to assess the performance and resilience of strongly interconnected systems.
In the process of identification of big data indicators, a bottom-up approach is required. It starts by harvesting existing indicators and identifying potentially relevant new indicators through data mining, e.g. using big data or open data sources.
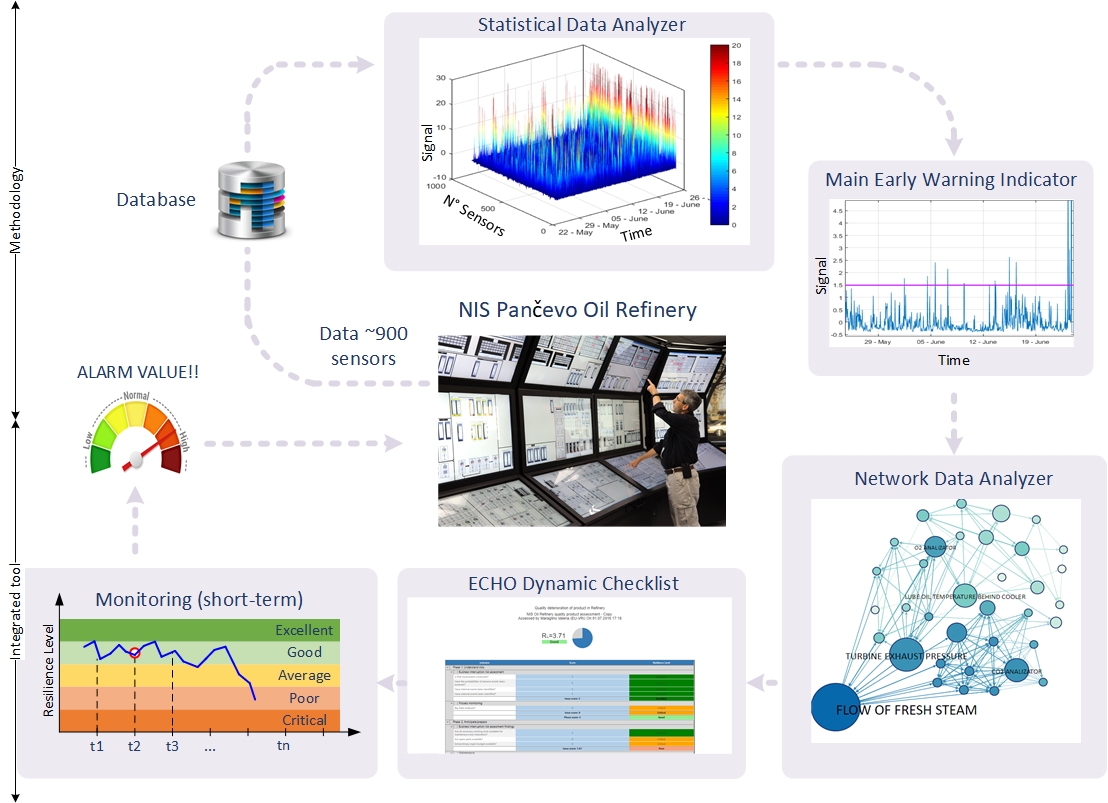
[Click on the pic to login SmartResilience page and load Dynamic Checklist ID 41 related to quality product assessment for ECHO]
City of Pančevo has the so-called Southern Industrial Zone located at the southeast edge of town, right next to the residential area of the city, app. 4 km away from the city center. In addition to the compound of the HIP-Petrohemija a.d. Pančevo, this zone includes the HIP Azotara Pančevo a.d. and NIS Oil Refinery Pančevo. The area is connected to road, rail and river circulation by means of the port on the Danube River. In this industrial zone there is a production of petroleum products, basic chemical products, polyethylenes, mineral fertilizers, calcium ammonium nitrate, carbamide and NPK fertilizers.
One of the big data set analyzed for the refinery is the time-series data of ~1,000 sensors in petrochemical plants. The end user provided time-stamped data from about 1,000 sensors installed at the NIS Oil Refinery. Each of these sensors provides a time-series of measured values that can be used to assess the performance of a specific component of the petrochemical plant. In particular, this data covers a timespan where an adverse event occurred that lead to a deterioration of the quality of the output of the plant.
Alarms are monitored over DCS (Distributed Control System). DCS is a computerized control system for a process or plant, in which autonomous controllers are distributed throughout the system, but there is central operator supervisory control. It increases reliability and reduces installation costs by localizing control functions near the process plant, but enables monitoring and supervisory control of the process remotely. It is used in process industry to provide high reliability and security.
The big amount of data pose a data relevance challenge. Here is developed a methodology to use large-scale data to identify and quantify resilience through a unified indicator of resilience capacity. A given set of input is ranked according to their potential to generate useful information as measured by the correlation among each other. This indicator can be used to prioritize, together with conventional indicators, investment, to measure progress over time in increasing resilience capacity and to provide performance measures of infrastructure systems to estimate the return of the investment of those measures. Here below the Resilience assessment of NIS Oil Refinery quality product.
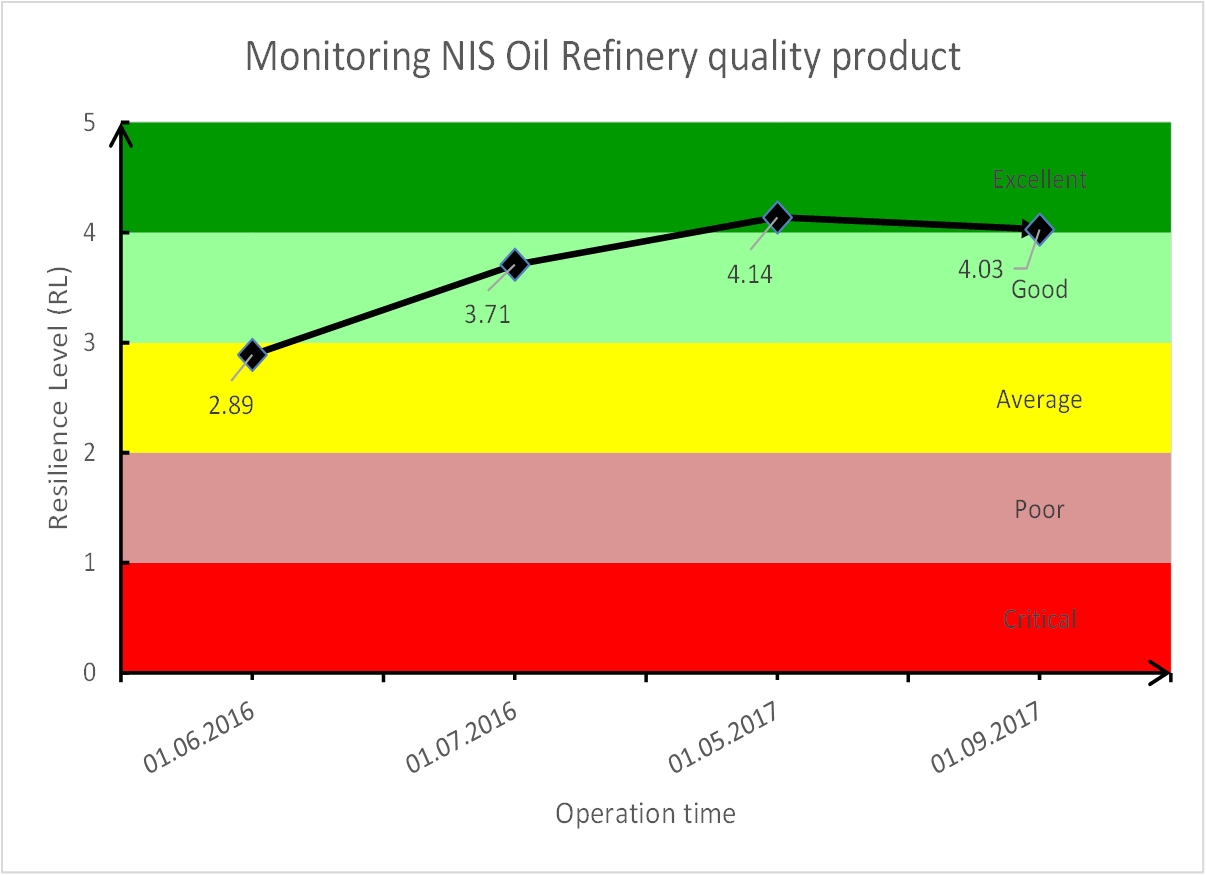
Assessing resilience of a SCI starts from assigning values to single indicators, which corresponds to scores on a five-level scale from 0-5, where 0 is worst and 5 is best. At each level, the scores – alternatively combined with weights – corresponds to a certain resilience level. The purpose of assessing resilience is to obtain a quantitative measure of how resilient an area such as a city or an individual SCI are against severe threats. This results show the level of resilience of NIS refinery over operation time and where improvements are most needed, emphasizing and fostering a continuous improvement mindset through regularly updated assessments.